5 Lexical relations
5.1 How do antonyms emerge?
We have seen quite a few examples of various types of antonymy in Justeson & Katz (1991). Let’s approach this from a different perspective by first thinking about synonyms, words with the same meaning, rather than the opposite. A typical example is the pair buy and purchase. Both words have to do with the exchange of money in one direction and the exchange of some sort of counter value in the other direction. Sameness can be claimed on the basis that substituting those words in context won’t change the proposition of the sentence.
- We’ve bought more of the chocolate you like.
- We’ve purchased more of the chocolate you like.
Roughly speaking, the real-world situation the sentences describe is likely the same. Yet the example in 23 sounds unusual in the context of chocolate. The substitution is also not possible in all contexts. Consider the following example where a different sense of buy is used that roughly means believe.
- She told me she’d never eaten chocolate, but I don’t buy it.
- She told me she’d never eaten chocolate, but I don’t purchase it.
The reading believe is not available for purchase. True synonymy in the sense of perfect substitutability is rare if it exists at all. The only candidates might be dialectal variations of words with very specific meanings, such as German Brötchen, Schrippe, Semmel. There are always differences in connotation and at the very least differences in use. You find different distributions, collocations and fixed expressions. For example, the phrase Brötchen verdienen is common in German but Schrippen verdienen isn’t, even in regions where Schrippe is the more common word.
At this point, the existence of true synonyms depends on the very definition of meaning. In Usage-Based linguistics, the lines between denotation, connotation and distributional properties is blurred on the assumption that all of these aspects are intertwined. The “Principle of no Synonymy” (Goldberg 1995) is a prominent idea from this paradigm. Meaning is seen as being inseparable from use, therefore, co-occurrence patterns become extremely important.
If lexemes cannot have exactly the same meaning, can they even have opposite meanings? Does it even make sense to speak of opposite use? There is clearly an intuition for oppositeness. There are two main ways to explain antonymy. The traditional one is that antonyms can paradigmatically replace their opposite.
Paradigmatic, replaceable
- He was a good dog.
- He was a bad dog.
- I feel good today.
- I feel bad today.
In fact, that makes them extremely similar in their use. You would expect similar collocates, constructions and syntax.
Justeson & Katz (1991) argue against this view and propose that the intuition we have that some words have direct opposites is grounded in their co-occurrence syntagmatically.
Syntagmatic, co-occurrence
- There are good and bad dogs.
- Some dogs are good, some are bad.
- I feel neither good nor bad.
- Good jokes make people laugh, unlike bad ones.
One fascinating aspect of these observations is that, while synonyms occur in wildly different contexts, antonyms tend to occur together. The hypothesis is that we think of antonyms as antonyms exactly because we see experience them together all the time. high and low are contiguous; they co-occur—high and flat are not, because they don’t not because of some objective meaning components. In fact, there is usually only one antonym within a set of synonyms. If we assume a componential model based on truth-conditions, this would be difficult to explain. Taking logical meaning components alone does not explain speaker intuition.
5.2 Causal relationships
The findings from Justeson & Katz (1991) work very well with cognitive concepts of memory and learning. An interesting interpretation of the findings would be that we don’t need any inherent meaning to explain antonyms. Children would just learn what antonyms are through language use. Here, we are falling for a common trap though, which is bias towards a specific theory. The findings might be consistent with more than one theory. In order to evaluate the usage-based interpretation, we should also consider alternative explanations.
Usage-based linguistics is most strongly contradicted by nativist theories, such as Universal Grammar. The idea of nativists is that we are born with a capacity for language including some deep undelying linguistic categories. In this view, children already have categories like antonymy (or more generally oppositeness) hard-wired in their brains. Language learning then would consist of categorizing new stimuli against these pre-existing categories. It would be possible to imagine that the structure for antonym relationship is already given and children learn which words are antonyms. As a result of that, use them together more often than other word pairs.
Ultimately, it is a question of causality. Did co-occurrence cause the emergence of antonym pairs, or did the oppositeness of the lexemes cause the co-occurrence? We have arrived at a chicken or egg situation:
- Antonyms co-occur and children/learners associate them. Their oppositeness is a result of this.
- Antonyms have opposite meanings, children learn that first and, as a result, use them together.
One of the main routes to take is the search for an a priori definition of antonymy or oppositeness that we would need to determine a potential pre-language concept. Observational methods are not normally used to infer causal relationships. This is normally the job of experimental methods, where you can setup a controlled environment for language to be produced in order to control for as many confounding variables as possible. However, when it comes to children, the options are limited and it is hardly possible to check whether there is a pre-language concept of antonymy. Without empirical data, the options are limited but, you can still theorize over the most likely explanations. Non-empirical methods are common in literary and cultural studies and also philosophy.
When it comes to language and children, there have been a number of stories and questionable unethical experiments. Friedrich II. and the Nazis experimented on children and deprived them of language, and there have been multiple accounts of orphaned children who grew up alone or with animals. It is tempting to take these stories as evidence. However, neither of these accounts have been carried out with the necessary academic rigor, and there was usually some sort of ideological agenda. Anecdotal evidence is often full of contradictions and fantasy. Sometimes the stories are distorted to fit a particular belief, and sometimes contradicting aspects are simply left out. For the most part, observations that are non-reproducible are not viable even if the reasons for their non-reproducibility are ethical.
In conclusion, many observations in corpus linguistics are simply evidence for correlations, and it is very hard to infer causal relationships without the help of experimental methods. If those are not available, it is necessary to have a good grasp on the philosophy of science to narrow down possible interpretations. In any case, you always have to be aware of the limitations of the data and methodology.
5.3 Co-occurrence/correlation/contiguity
Correlation is a concept that should be common knowledge. It is most commonly encountered in the context of statistics. Roughly speaking a correlation is a close numeric relationship between data points. The most common type of correlation we encounter in corpus linguistics is co-occurrence, which is simply the observation that two linguistic structures happen in the same environment, mostly the same text, sentence, phrase or even right after one another. Another con word we have encountered is contiguity. Same prefix (con, Latin for with, together) same general idea, but different context. Contiguity is most often used in the sense of co-occurrence on the level of experience. It stresses the psychological aspects of perception and memory and what is perceived as occurring together. Contiguous stimuli aren’t necessarily correlated from an objective point of view. The main contrast to association that arises from contiguity is association that arises through similarity.
Consider the following scenario:
Every time you leave your house, an elderly woman from next door screams God bless, out of her window. It is likely that you will be reminded of this grandma when you hear this phrase. You associate the two because there is temporal contiguity. You might likewise be reminded of the woman and the phrase when you leave your house and the old lady is not around because there is also local contiguity.
Statistically speaking, there is no correlation between old women and yelling God bless out of windows. On a broader scale, however, the phrase might be correlated, i.e. more common, with older speakers. The correlation might be weak, but it isn’t unreasonable to hypothesize that. In order to judge that, only one piece of evidence alone isn’t enough to establish a correlation.
Now, on a linguistic level, there is definitely a relationship between God and bless. A linguistic con concept (no pun intended) is collocation, which refers to words that occur together significantly often. On the level of the individual piece of data, which is you experiencing this phrase again and again, in this particular discourse situation, the two words simply co-occur. Co-occurrence is just when things happen at the same time and/or same place. In order to know whether God bless is a collocation, you’d need more evidence for both God and bless.
All these differences might be subtle, but they should rarely cause confusion. The biggest differences lies in the communicative context they are used in. In some sense, the relationship between some of those con words is one of synonymy, with which we have come full circle for this week. Them being very similar doesn’t mean, however, that they are interchangeable, especially not in academic prose.
If anything, the principle of no synonymy is even truer in academic language. ;)
5.4 Reproducing Justeson & Katz 1991
Using CQP and the Brown Corpus, which is available on our server, we can try to reproduce the results. If you want to look at a pair in detail, you can do the following:
BROWN;
old = [word = "old" %c & pos = "JJ.*"] expand to s;
young = [word = "young" %c & pos = "JJ.*"] expand to s;
young_and_old = intersect young old;
size young;
size old;
size young_and_old;
Here are all commands in detail:
-
BROWN
— activate the Brown Corpus -
old = ...
— binds the query results to a variable calledold
-
[...]
— look for one token -
[word = "old" ...
— match the orthographic form old -
%c
— ignore case, i.e. also search for Old, OLD, oLD, etc. -
... pos = "JJ"]
— also match the pos-tag JJ which stands for adjective (see cheatsheet or type info in CQP) -
expand to s
— match the entire sentence surrounding -
intersect young old
— keep all matches that include those from young and old (intersection = Schnittmenge) -
size
— print number of matches (absolute frequency) -
;
— end command; this is only necessary if run as a script; it is otherwise equivalent to hitting enter
In summary, this searches for all sentential co-occurrences in Justeson & Katz (1991), i.e. old and young within the same sentence.
Of course, if we want to do this for all adjectives from the paper, this is too tedious. We can instead find all adjective pairs within the same sentence.
adj = [pos = "JJ"] []* [pos = "JJ"] within s;
set PrettyPrint no;
group adj match lemma by matchend lemma > "coocurrences.csv";
all = [pos = "JJ"];
group all match lemma > "all.csv"
This looks for adjectives within a sentence with anything in between, and a frequency list of it to a file. We also create a second file with a frequency list of adjectives.
-
[]*
— a token without constraints, so any token, and it can be repeated 0 times or any amount of times. -
within s
— the match needs to be within a sentence, so[]*
cannot cross sentence boundaries -
set PrettyPrint no
— removes some formatting from the output so that we can work with it more easily later -
group
— make a frequency list (similar to count) -
all
— name of our variable holding results of[pos = "JJ"]
-
match lemma
— count the lemma of the first token of the match (equivalent tomatch[0] lemma
) -
>
— redirect output -
"..."
— file name to redirect to; in our case, all.csv and coocurrences.csv
For further processing, CQP is not the right tool anymore. The next step would be to filter out some combinations that we are not interested in and find the Deese antonyms etc. This is a job for scripting tools like R, Python or Excel, and we’ll go over it in the future.
5.5 Collocation
Major Concepts
- Collocation and Co-occurrence
- Frequency and relative frequency
- Probabilistic properties of language
5.6 Quantities in corpora
In some sense Corpus Linguistics is really simple since it is largely focused on one basic indicator: frequency of occurrence. All annotations, such as lemma, pos, text type, etc. are ultimately measured in counts. There are hardly any other types of indicators, such as temperature, color, velocity, or volume. Even word and sentence lengths, are fundamentally also counts (of characters/phonemes, or tokens/words).
This simplicity, however, comes at a cost. Namely the interpretation is rarely straightforward. We’ve talked about how ratios become important if you are working with differently sized samples. I use sample here very broadly. You can think of different corpora as different samples, but even within the same corpus, it depends on what you look at. Let’s assume we were investigating the use of the amplifier adverb utterly. As a first exploration, we might be interested whether there are some obvious differences between British and American usage. For the British corpus, let’s take the BNC (The British National Corpus 2007), and for the American corpus the COCA (Davies 2008).
> cqp
BNC; utterly = "utterly" %c
COCA-S; utterly = "utterly" %c
size BNC:utterly; size COCA-S:utterly
...
1204
4300
The American sample returns more matches. Does that mean it is more common? No! The American sample is also about 5 times larger. We need to account for the different sizes by normalizing the counts. The simplest way to achieve that is by calculating a simple ratio, or relative frequency.
- \(\frac{1248}{112156361} = 0.0000111\)
- \(\frac{4401}{542341719} = 0.0000081\)
Now we can see that utterly actually scores higher in the British sample. We can make those small numbers a bit prettier by turning them into occurrences per hundred a.k.a percentages, which gives us 0.00107% and 0.00079%. That’s still not intuitive so let’s move the decimal point all the way into intuitive territory by taking it per million. utterly occurs 11.1 times per million words in the British sample and 8.1 times in the American sample.
Now how about the nouns utterly co-occurs with. To keep it simple, let’s focus on the British data.
BNC
count utterly by word on match[1]
...
34 and [#91-#124]
28 . [#20-#47]
27 different [#408-#434]
20 , [#0-#19]
13 at [#142-#154]
13 ridiculous [#929-#941]
11 impossible [#645-#655]
11 without [#1207-#1217]
10 miserable [#772-#781]
10 opposed [#820-#829]
The two most frequently co-occurring adjectives are different and ridiculous. However, different is more than twice as frequent. Does that mean it is more strongly associated? No! The adjective different is itself also much more frequent than ridiculous (~47500 vs 1777).
5.7 Perception of quantities
Relative frequency and other ratios are not only interesting when you need to adjust for different sized samples. Human perception is also based on ratios, not absolute values. In some sense our brains also treat experiences on a sample-wise basis. Of course, you would not normally speak of experience as coming in samples, but remember the ideas of contiguity and similarity. We know that some of the structure in memory, therefore, structure in language, comes from spatially and temporally contiguous experiences, and/or the degree of similarity to earlier experiences. But of course we don’t remember every word and every phrase in the same way. Repetition and exposure to a specific structure play a key role. Now that we have a little experience with assessing what is relatively frequent in linguistic data, we should start thinking about what is frequent in our perception. An observation that has repeatedly been made in experiments is that absolute differences in the frequency of stimuli become exponentially less informative. This is captured by the Weber-Fechner law.
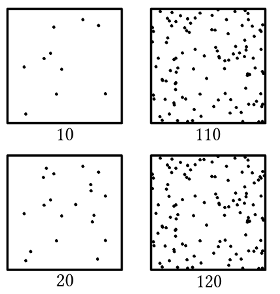
The observation is that the difference between 10 and 20 items is perceived as much larger than the difference between 110 and 120 items, even though they are the same in absolute terms. The higher the frequency of items, e.g. words, the higher the difference to another set has to be in order to be perceivable. This is strongly connected to the exponential properties of Zipf’s Law, which we will talk about in Week 7 and 8.
5.8 Collocation as probabilistic phenomenon
Collocations are co-occurrences that are perceived and memorized as connected. Some collocations are stronger, some weaker, and some are so strong that one or all elements only occur together, making it an idiom or fixed expression.
- spoils of war: idiom
- declare a war: strong collocation
- fight a war: weak collocation
- describe a war: not a collocation
- have a war: unlikely combination
- the a war: ungrammatical
The above examples are ordered according to the probability that they can be observed. We will get into the details of how we can quantify this exactly in the lecture and in future seminar classes. If you are impatient, www.collocations.de has a very detailed guide to how that works. For now, the logic is simply that we consider not only the different frequencies of the individual phrases, as in the examples above, but also the frequencies of the each word in the phrases, the words in the corpus, and all attested combinations of [] a war.
The important takeaway is that a defining property of collocation is gradience. That means that it is a matter of degree. The more frequent, a collocation is, relatively speaking, the more likely it is to be memorized as a unit. It is not a question of either or, or black and white. This idea has since been described for most if not all linguistic phenomena, including grammatical constructions, compound nouns, and even word classes, just to name a few.
5.9 Homework
If you haven’t done so, catch up with the past homework. Watch the tutorial and get your hands dirty with the examples in this article.
- Watch §4 through §6 from this CQP tutorial: CQP Playlist
- Think about morphological antonyms (e.g. convenient vs inconvenient, wise vs unwise)
- what prefixes create antonyms? are there suffixes too?
- how regular are those affixed words, do have notable exceptions?
- Pick an affix and try to search for it, using techniques from this blog or the YouTube tutorial.
5.9.1 Tip of the day
Use spreadsheets! You will inevitably have to at some point enter some numbers into something like LibreCalc, Microsoft Excel, or Google Sheets. We will benefit from spreadsheets throughout this module, but this is not where their utility stops. Being able to do some quick formulae and vlookups in Excel are common skills needed outside Uni.
Especially for teachers, spreadsheets are an essential skill: for grades, averages, homework, quick stats on exams, lesson planning, Sitzplan (oh memories :D), what have you. If you know your way around Excel, you can speed up your tax returns (Steuererklärung) a lot, too. Many teachers end up working as freelancers. For a freelancer (and anyone else really), gathering your receipts, bills and pay slips neatly arranged and categorized as data in a spreadsheet can save you endless amounts of time and even money.
This is not where it stops though. Timetables and To-Do-Lists are also neat to do in a spreadsheet if you need more fine-grained control over the layout than the clunky online calendar you are probably using. Here are some things I have used spreadsheets for in the past: notes, training log, travel plans, shopping lists. You could even use them for recipes or counting calories if that’s what you’re into.
I myself have since moved past Excel/Calc and use only plain text files. If I need to do some maths or stats, I use .csv or .tsv files in combination with statistical software such as R. That might seem to be the ultra-nerd level, but isn’t so difficult to learn at all, and can save you additional time and frustration. Maintaining a CV for example is a breeze if you have everything as plain data and deal with the formatting in an automated fashion, and only when you need to.